Adopting AI in the Enterprise: Ford Motor Company
Check out the Strata Business Summit at the Strata Data Conference in New York City, Sept. 25–28, 2017, to learn more from data-driven…
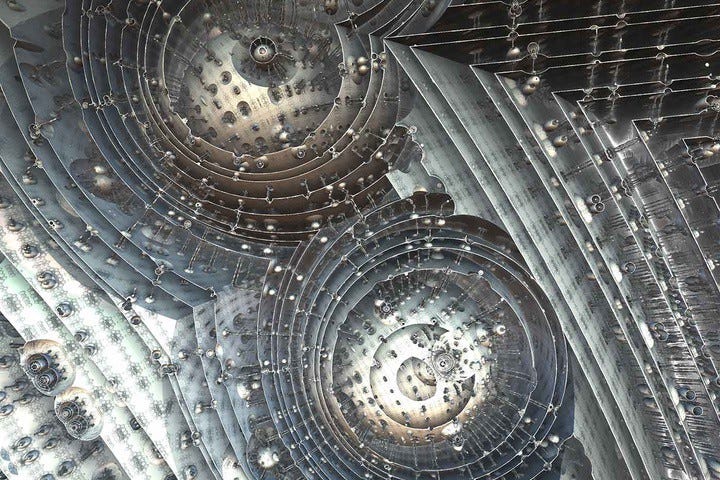
Check out the Strata Business Summit at the Strata Data Conference in New York City, Sept. 25–28, 2017, to learn more from data-driven businesses — including American Express, BBC Worldwide, and LinkedIn. Early price ends August 11.
Driverless cars aren’t the only application for deep learning on the road: neural networks have begun to make their way into every corner of the automotive industry, from supply-chain management to engine controllers.
In this installment of our ongoing series on artificial intelligence (AI) and machine learning (ML) in the enterprise, we speak with Dimitar Filev, executive technical leader at Ford Research & Advanced Engineering, who leads the team focused on control methods and computational intelligence.
What was the first application of AI and ML at Ford?
Ford research lab has been conducting systematic research on computational intelligence — one of the branches of AI — for more than 20 years. About 15 years ago, Ford Motor Company introduced one of the first large-scale industrial applications of neural networks. Ford researchers developed and implemented, in mass-produced cars, an innovative misfire detection system — a neural-net-based classifier of crankshaft acceleration patterns for diagnosing engine misfire (undesirable combustion failure that has a negative impact on performance and emissions). Multiple other AI applications to Ford product and manufacturing followed this success.
How do you leverage AI and ML today to create a better product?
We can think of two categories of ML and AI applications in our vehicles. In addition to the obvious applications in driverless cars, Ford has also developed AI-based technologies that enable different functions in support of vehicle engineering. These are not always visible to the driver.
As I mentioned before, we used recurrent-neural-net-based classifiers for misfire detection in V10 engines; we also use them for intruder detection when the driver is away from vehicle. We also use fuzzy logic-type rule-based gain scheduling controllers integrated with the battery control systems of hybrid-electric vehicles.
In our supply chain, neural networks are the main drivers behind the inventory management system recommending specific vehicle configurations to dealers, and evolutionary computing algorithms (in conjunction with dynamic semantic network-based expert systems) are deployed in support of resource management in assembly plants.
Are there other use cases within Ford today?
Another group of AI applications is driven by the fact that current vehicles have evolved into complex mobile cyber systems with increasing computational power and resources generating gigabytes of information per hour, continuously connected, with information following to, from, and through the platform. Increased capability of vehicle systems, along with the growing customer demand for new features, product improvement, personalization, rich information utilization, etc., are some of the drivers for introducing machine learning techniques in modern vehicles.
The most common AI applications involve direct driver interaction, including advisory systems that monitor acceleration and braking patterns to provide on-board evaluations of a driver’s preferences and intentions for different purposes — characterization of the driver, advice for fuel-efficient driving and safe driving, auto-selecting the optimal suspension and steering modes, simplifying the human-machine interface by estimating the most likely next destination, and preferred settings of the climate control, etc. These systems use traditional AI methods — rule-based, Markov models, clustering; they do not require special hardware. One of their distinctive features is to be intelligent enough to identify the level of acceptance of provided recommendations, and avoid drivers’ annoyance.
Recent extensive development of autonomous vehicles is the driver for deep learning applications to vehicle localization, object detection, classification, and tracking. We can expect in the near future a wide range of novel deep-learning-based features and user experiences in our cars and trucks, innovative mobility solutions, and intelligent automation systems in our manufacturing plants.
What steps have you needed to take to build a team that can grasp and apply recent advances in AI and ML?
We have several centers of excellence in machine learning and AI, with focus on robotics, next-generation autonomous driving, and data analytics. Our goal is to expand the AI-based methodology and development tools throughout the company and to make them part of the commonly used engineering tools, similar to Matlab and Simulink.
Building centers of excellence in AI and ML was not too challenging since, as I mentioned earlier, we had engineers and researchers with backgrounds and experience in conventional neural networks, fuzzy logic, expert systems, Markov decision processes, evolutionary computing, and other main areas of computational intelligence. This created the foundation that we are now upgrading with a state-of-the-art expertise in deep learning methods and tools. We continue to expand this critical mass of experienced engineers by hiring more computer specialists with strong educational backgrounds in AI and ML.
Where does Ford hope to gain a competitive advantage in applying AI and ML?
AI provides an opportunity to better use available information for creating new features and driver-aware, personalized vehicles that would better fit to the specific customer. In addition, machine learning is an irreplaceable enabler for creating smart driver-assist systems and fully autonomous vehicles. Increased connectivity is one of the major drivers expanding the capability of the on-board infotainment and control systems by incorporating cloud resources. In the near future, we can envision seamless integration of vehicle on-board systems with cloud-based intelligent agents, self-organizing algorithms, and other AI tools that would broaden the range of user experiences offered by our mobility solutions.
Are there any areas where you’ve considered leveraging AI/ML but found that the technology isn’t ready yet?
I don’t think so — just the opposite. It seems that the AI/ML toolbox is growing exponentially and ahead of mass applications. We are witnessing an interesting reality — while many research areas (e.g., control engineering, computer programming, cybernetics) were driven by the need for new technical solutions, the AI revolution that is happening now is inspired by the advances in machine learning research. Besides the stimulating effect of some remarkable successes (e.g., Google DeepMind), I would like to mention two important and unique enablers of this rapid development — first, the quick proliferation of research ideas and results that are made immediately available by posting recent publications on arxiv.org or other public websites; and second, the wide accessibility to open source AI software development tools — TensorFlow, Neon, Torch, Digits, Theano, just to mention a few. The challenge now is to mature the most effective and innovative AI solutions, and to integrate them within new features and customer experiences.
Is Ford interested in partnering with other Silicon Valley companies and startups? Are there any initiatives you’d like to see the community focus on?
Ford Motor Company has partnerships with a number of high-tech companies and startups around the world, and, of course, in Silicon Valley. We are an active member of the innovative Silicon Valley community through our Research & Innovation Center in Palo Alto and are always interested in working with new companies and startups.
What’s the most promising or interesting advancement you’ve seen in AI recently, and how do you think it will impact Ford?
It is hard to outline the most interesting one, for the progress in AI is enormous. The number of publications, patents, and software products in the AI area is exponentially growing, and almost every day we are witnessing new accomplishments, novel approaches, and smart applications. I am specifically interested in the developments in reinforcement learning, intelligent agents, game theory, and Markov decision processes since they open the door to new advancements in the field of automated reasoning, decision-making and optimal control, and their automotive and mobility applications.
Originally published at www.oreilly.com on July 20, 2017.